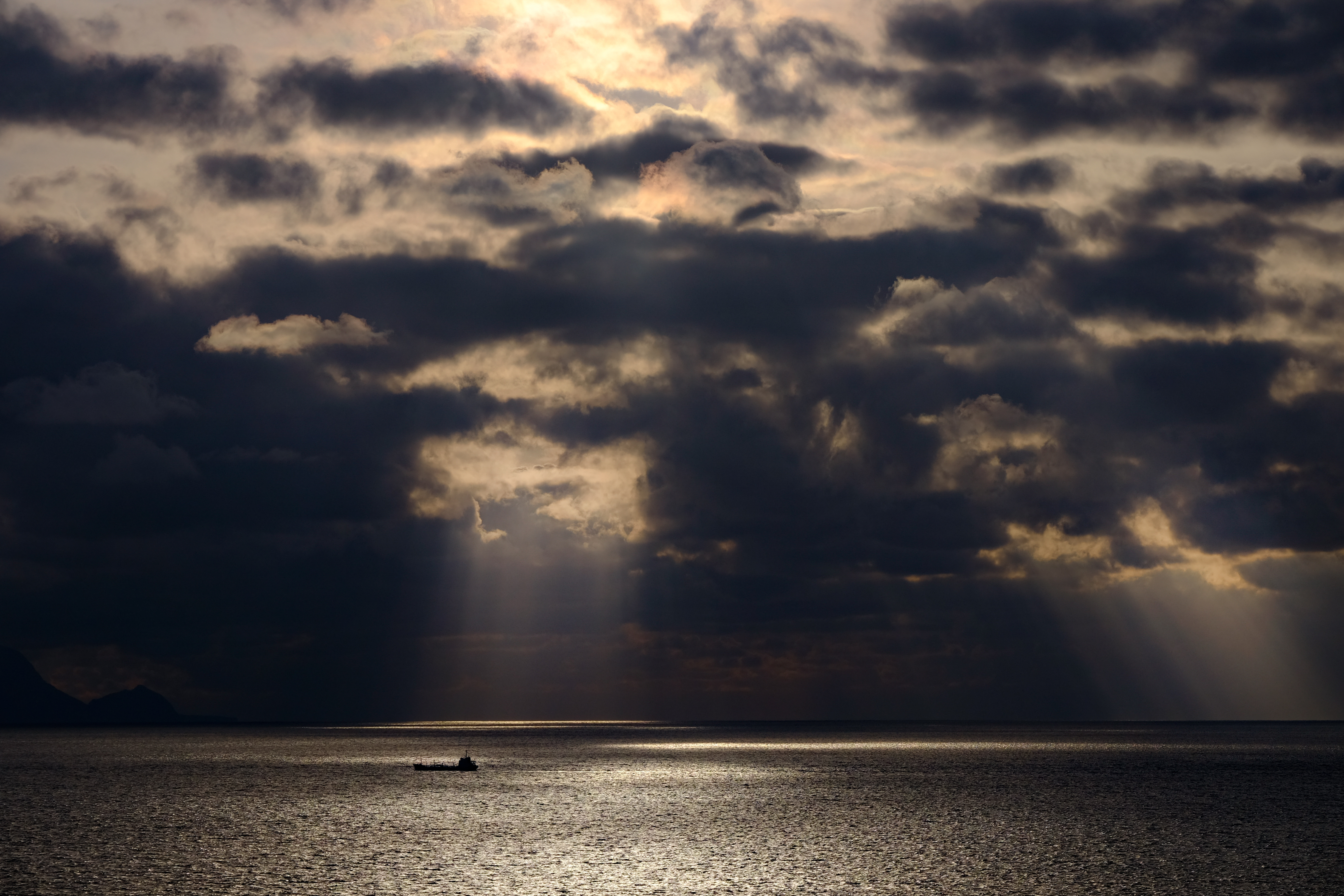
In a sea of data - How companies can create value from their data
Nearly all relevant business decisions are based on data. But in this rapidly growing flood of information lies both an immense opportunity and a potential danger: If this data is not managed properly, it can be misleading and useless. According to a study by Forrester, up to 73% of data in an organization is never used strategically, and research by Carnegie Mellon University found that 90% of data in an organization is unused data or "dark data". In order to gain value from data, it is necessary for it to be meaningful and reliable in order to serve as a reliable basis for decision-making.
Data quality as a breakwater
The amount and importance of data for businesses is growing every day. However, simply capturing and storing data does not make it a valuable asset, nor does it make a company data-driven. Data is specifically useful when it is captured and managed with a defined purpose so that the company has high quality data to make important business decisions. Otherwise, there is a risk of not being able to rely on the data. Poor quality data can lead to inaccurate analysis and poor decisions, negatively impacting business performance.
From a business perspective, data quality is about ensuring that data is suitable for its intended use in operations, decision making, and planning, and that it meets internal and external compliance requirements. With high-quality data, companies can accelerate decision-making processes, define and implement targeted activities, and produce high-quality forecasts for business development.
Quality data not only helps to increase the efficiency of the data inventory, but also helps to minimize risks, reduce costs and increase productivity. Data managers spend less time checking interfaces, analyzing errors and can instead focus more on using the data for their purposes.
Consequently, it is necessary to define the purpose at an early stage, i.e., which decisions are to be supported by data. Data and information as well as their quality are thus becoming a decisive factor - also in terms of competition - to which companies should attach central importance. For this reason, it is also advisable to introduce a company-wide data management system that overcomes the historical IT silo mentality and can act cross-sectionally.
Data governance and data management: port and starboard of data quality
Data governance and data management go hand in hand in the realization of data quality, with the former focusing on the regulatory framework and policies and the latter on procedures as well as processes.
Data governance policies define cross-organizational roles and responsibilities as well as associated decision rights and guardrails for orderly control of data management.
To this end, the data governance team works with the relevant stakeholders to define the criteria and procedures for the collection and quality of the data.
Data management is about data throughout its lifecycle within an organization. An end-to-end data management strategy can help ensure that data is managed throughout the process - from creation to deletion - in accordance with regulatory and industry requirements.
A data governance policy defines, among other things, that the collection and management of data complies with the GDPR-based requirements and thus creates the bridge between the regulatory requirement and the company-specific implementation.
According to a study by McKinsey, data governance is a crucial success factor for companies. However, companies sometimes do not attach enough importance to this topic, which can lead to high costs and - among other things - regulatory risks. Without quality-assured data management, companies not only miss out on opportunities arising from data-driven opportunities, but also waste valuable resources. According to the study, processing and cleansing data can take up more than half the time of a -well-paid- analytics team. This limits value-added work and frustrates employees. In fact, employee productivity across the organization can suffer: In a 2019 survey, participating respondents indicated that poor data quality and availability caused an average of 30 percent of their total company time to be spent on non-value-added tasks.
All hands on deck! - Data quality as a shared responsibility
Realizing data quality is a continuous process that should involve the entire organization. In many companies, the process is primarily understood as a pure technology project, but this goes beyond the mere technical integration of data. Before integration into a system, it is crucial that those responsible develop a uniform understanding of the role that the various data points can play in the company's value chain.
Improving data quality should be a high priority for business leaders, as effective data management and governance has a significant impact on business decisions.
The strategy for defining, collecting, checking, using and deleting data in companies is increasingly becoming a decisive factor in competition. For this reason, there should be a uniform understanding of what, how, and for what purpose data management is used, starting with the management and extending to the IT organizations. Only an integrated process and its implementation can ensure that the necessary data is available in the appropriate form at the right time.
Author: Cüneyt Baluch